#data science in finance
Explore tagged Tumblr posts
Text
Discover powerful applications of Data Science in Finance that are transforming FinTech, from fraud detection to personalized services and algorithmic trading
0 notes
Text
0 notes
Text
Tinkering with my personal website again
Above screenie is zoomed out to capture everything. Anyone wanna guess which blinkies I made? Also, the Twitter blinkie just takes you to my BSky lol (on purpose).
Several of the images were put together by me! I can teach pretty much anything in tech, this is just the stuff that I thought of.
I made the floppy-disk icons myself, with some help from wifey on getting the text to render as part of the SVGs!
#personal website#web development#vaporwave#love2d#pico8#personal finance#tutoring#math tutoring#math teacher#computer science#computer science tutoring#game development#electronics design#pcb design#learntocode#machine learning#artificial intelligence#ai#ai development#data science#unity engine#godot engine
2 notes
·
View notes
Text
The mission of the Gordon Ford College of Business is to actively support and engage students, faculty, staff, and community in academic and lifelong professional development, providing the foundation needed to lead in today's diverse business environment.
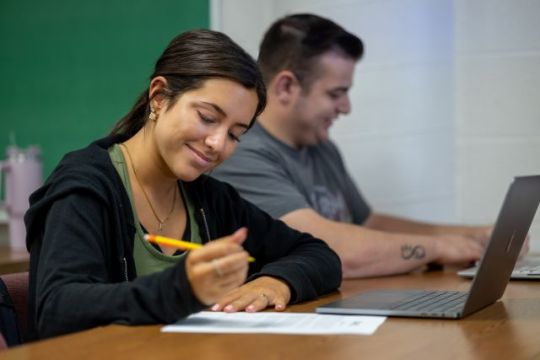
#supply chain#Sales#Marketing#management#Human Resources#Financial Planning#finance#economics and business analytics#economics#data analytics#cybersecurity#business data analytics#actuarial sciences#Accounting#Business
3 notes
·
View notes
Text
Top 10 PGDM Colleges in Mumbai | VSM
Valia School of Management is the Top 10 PGDM Colleges in Mumbai, offers a 2-year, full-time Post Graduate Diploma in Management (PGDM). With this AICTE-approved program, you will acquire hands-on experience toward industry excellence. With specializations in Marketing, Finance, Human Resources, Artificial Intelligence, and Data Science, our curriculum emphasizes practical learning through rigorous case studies and valuable guest sessions led by industry experts. Join Top 10 PGDM college in Mumbai to experience one of the top PGDM programs and launch your journey to success.
#PGDM#pgdmprogram#managementschool#mumbai#valiaschoolofmanagement#Top business school in Mumbai#top pgdm college in Mumbai#marketing#finance#human resources#artificial intelligence#data science#pgdm program#pgdm colleges#pgdm career
1 note
·
View note
Text
busy busy fox
these past couple of days I've been working my way through (thus far) the first 3 problem sets for MIT OCW 6.0001 and the first part of the Lean game, getting my github squared away to have a repo+folder system for a coding portfolio
kind of a long shot, I know, but if any of you reading this (yes! you! even though you don't think it'll make a difference!) want to pass me on as a reference for SWE/datasci/quant finance jobs... please please do? I really need to get hired somewhere??? relocation is not an issue and if anything it's ideal! hopefully I end up in Chicago or SF or Seattle but I am not actually that picky.
or if you have some advice or words of kindness please DM! I really really do not want to have to give up and grind myself into a paste in the Precalc Adjunct Crusher!
frankly I'm kinda frustrated with how much of the wall out of academia I've been trying to climb for the last couple of years has been industry... not wanting people without prior work experience, unless they're interns, and they don't offer internships to postdocs regardless of the whole "2020-2022 was just plain fucked for internships" thing. which is a shame because that is when I graduated!
so please: help me get a tech job of some kind so that I can start functioning at my fullest again!
#foxoftindalos#due to my agonies#data science#software engineering#coding#computer science#quantitative finance
0 notes
Text
How AI Identifies Emerging Market Opportunities in Financial Analysis
In the fast-paced world of finance, identifying emerging market opportunities is crucial for investors, analysts, and businesses looking to maintain a competitive edge. Traditional methods of market analysis often involve extensive manual research and interpretation of data, which can be time-consuming and prone to human error. However, the advent of Artificial Intelligence (AI) is revolutionizing financial analysis by enabling quicker, more accurate identification of market trends and opportunities. Photon Insights is at the forefront of this transformation, leveraging AI to help financial professionals uncover valuable insights. This article explores how AI is reshaping the landscape of financial analysis and the role of Photon Insights in this evolution.
The Importance of Identifying Emerging Market Opportunities
Identifying emerging market opportunities is vital for several reasons:
1. Strategic Investment Decisions: Investors rely on accurate market analysis to make informed decisions about where to allocate resources. Recognizing trends early can lead to significant financial gains.
2. Competitive Advantage: Businesses that can spot emerging markets ahead of their competitors can position themselves effectively, capturing market share and driving growth.
3. Risk Mitigation: Understanding market dynamics allows firms to anticipate changes and potential risks, enabling proactive strategies to safeguard investments.
4. Innovation and Growth: Emerging markets often present unique opportunities for innovation. Identifying these trends can inspire new products, services, and business models.
Challenges in Traditional Financial Analysis
Traditional financial analysis methods face several challenges:
1. Data Overload: The sheer volume of data generated in financial markets can be overwhelming. Analysts often struggle to sift through this information to identify relevant trends.
2. Time Constraints: Financial professionals are under constant pressure to deliver insights quickly. Manual analysis can lead to delays in decision-making.
3. Subjectivity: Human bias can affect interpretation of data, leading to inconsistent conclusions and potentially flawed investment decisions.
4. Inability to Predict Trends: Traditional analysis often relies on historical data, which may not adequately reflect future market conditions.
How AI Transforms Financial Analysis
AI is redefining financial analysis by offering tools and techniques that address the challenges of traditional methods. Here are several key ways AI enhances the identification of emerging market opportunities:
1. Advanced Data Analytics
AI algorithms can process vast datasets from multiple sources, including financial reports, news articles, social media, and market data, in real-time. This capability allows analysts to identify patterns and trends that may not be evident through manual analysis.
Keyword Focus: Data Analytics, Market Trends
Photon Insights utilizes advanced data analytics tools to enable financial professionals to uncover insights quickly, facilitating timely investment decisions.
2. Predictive Analytics
By employing machine learning algorithms, AI can analyze historical data and identify factors that influence market movements. Predictive analytics can forecast potential future trends, helping analysts anticipate emerging opportunities.
Keyword Focus: Predictive Analytics, Forecasting
Photon Insights provides predictive analytics capabilities that allow financial analysts to simulate different market scenarios, enhancing their ability to make informed decisions.
3. Natural Language Processing (NLP)
NLP enables AI to interpret and analyze unstructured data, such as news articles and social media posts. By gauging public sentiment and market discussions, AI can help analysts understand market perceptions and identify emerging trends.
Keyword Focus: Natural Language Processing, Sentiment Analysis
Photon Insights integrates NLP features that help analysts gauge market sentiment, allowing them to make more nuanced assessments of market conditions.
4. Real-Time Monitoring
AI tools can continuously monitor market data, news, and social media in real-time, providing analysts with instant alerts about significant market changes. This immediacy enables prompt action in response to emerging opportunities.
With Photon Insights, financial professionals can set up customized alerts to stay informed about critical developments that may signal new market opportunities.
5. Enhanced Visualization
AI-driven data visualization tools can present complex datasets in an easily digestible format. Visual representations of data help analysts quickly identify patterns, correlations, and outliers, facilitating faster decision-making.
Photon Insights offers advanced visualization capabilities, enabling analysts to create interactive dashboards that highlight emerging trends and opportunities.
The Photon Insights Advantage
Photon Insights stands out in the field of financial analysis by providing a comprehensive suite of AI-driven tools designed to identify emerging market opportunities. Here are several key features of the platform:
1. Comprehensive Data Integration
Photon Insights aggregates data from various sources, including market data, news, and social media, providing a holistic view of market conditions. This integration enables analysts to identify emerging opportunities based on a wide array of information.
2. User-Friendly Interface
The platform features an intuitive user interface that simplifies the process of data analysis. Financial professionals can easily navigate the tools, making it accessible even for those with limited technical expertise.
3. Customizable Dashboards
Users can create customized dashboards tailored to their specific research needs. This flexibility allows analysts to focus on the most relevant data and visualizations for their market analysis.
4. Collaboration Tools
Photon Insights promotes collaboration among team members by allowing them to share insights and findings within the platform. This collaborative environment fosters a more comprehensive understanding of market dynamics.
5. Continuous Learning
The AI algorithms used in Photon Insights continually learn from new data, improving their accuracy and predictive capabilities over time. This continuous learning ensures that analysts are equipped with the latest insights and trends.
Case Study 1 — Investment Firm
One investment firm utilized Photon Insights’ NLP capabilities to assess market sentiment around a newly emerging technology sector. By quickly recognizing positive sentiment trends, timely investments were made in emerging tech startups with positive sentiment scores; as a result, this firm achieved exceptional returns from its investments.
Case Study 2 — Retail Business
One retail business used Photon Insights to keep up-to-date on consumer trends and preferences in real-time. By analyzing social media discussions and market data, they identified an increasing demand for sustainable products that allowed them to switch up their offerings and capture an attractive market niche.
Case 3 — Financial Services Company
One financial services firm utilized Photon Insights’ predictive analytics capabilities to anticipate any market disruptions caused by regulatory changes and develop proactive strategies for mitigating risks and seizing emerging opportunities in compliance-related services.
Conclusion
AI is revolutionizing financial analysis, helping professionals to recognize market opportunities faster and with greater accuracy than ever before. Photon Insights is leading this charge with its suite of AI-powered tools designed to enhance data analysis, predictive modeling, sentiment analysis, real-time monitoring and real-time alerts.
0 notes
Text
Making it easier to verify an AI model’s responses
New Post has been published on https://thedigitalinsider.com/making-it-easier-to-verify-an-ai-models-responses/
Making it easier to verify an AI model’s responses


Despite their impressive capabilities, large language models are far from perfect. These artificial intelligence models sometimes “hallucinate” by generating incorrect or unsupported information in response to a query.
Due to this hallucination problem, an LLM’s responses are often verified by human fact-checkers, especially if a model is deployed in a high-stakes setting like health care or finance. However, validation processes typically require people to read through long documents cited by the model, a task so onerous and error-prone it may prevent some users from deploying generative AI models in the first place.
To help human validators, MIT researchers created a user-friendly system that enables people to verify an LLM’s responses much more quickly. With this tool, called SymGen, an LLM generates responses with citations that point directly to the place in a source document, such as a given cell in a database.
Users hover over highlighted portions of its text response to see data the model used to generate that specific word or phrase. At the same time, the unhighlighted portions show users which phrases need additional attention to check and verify.
“We give people the ability to selectively focus on parts of the text they need to be more worried about. In the end, SymGen can give people higher confidence in a model’s responses because they can easily take a closer look to ensure that the information is verified,” says Shannon Shen, an electrical engineering and computer science graduate student and co-lead author of a paper on SymGen.
Through a user study, Shen and his collaborators found that SymGen sped up verification time by about 20 percent, compared to manual procedures. By making it faster and easier for humans to validate model outputs, SymGen could help people identify errors in LLMs deployed in a variety of real-world situations, from generating clinical notes to summarizing financial market reports.
Shen is joined on the paper by co-lead author and fellow EECS graduate student Lucas Torroba Hennigen; EECS graduate student Aniruddha “Ani” Nrusimha; Bernhard Gapp, president of the Good Data Initiative; and senior authors David Sontag, a professor of EECS, a member of the MIT Jameel Clinic, and the leader of the Clinical Machine Learning Group of the Computer Science and Artificial Intelligence Laboratory (CSAIL); and Yoon Kim, an assistant professor of EECS and a member of CSAIL. The research was recently presented at the Conference on Language Modeling.
Symbolic references
To aid in validation, many LLMs are designed to generate citations, which point to external documents, along with their language-based responses so users can check them. However, these verification systems are usually designed as an afterthought, without considering the effort it takes for people to sift through numerous citations, Shen says.
“Generative AI is intended to reduce the user’s time to complete a task. If you need to spend hours reading through all these documents to verify the model is saying something reasonable, then it’s less helpful to have the generations in practice,” Shen says.
The researchers approached the validation problem from the perspective of the humans who will do the work.
A SymGen user first provides the LLM with data it can reference in its response, such as a table that contains statistics from a basketball game. Then, rather than immediately asking the model to complete a task, like generating a game summary from those data, the researchers perform an intermediate step. They prompt the model to generate its response in a symbolic form.
With this prompt, every time the model wants to cite words in its response, it must write the specific cell from the data table that contains the information it is referencing. For instance, if the model wants to cite the phrase “Portland Trailblazers” in its response, it would replace that text with the cell name in the data table that contains those words.
“Because we have this intermediate step that has the text in a symbolic format, we are able to have really fine-grained references. We can say, for every single span of text in the output, this is exactly where in the data it corresponds to,” Torroba Hennigen says.
SymGen then resolves each reference using a rule-based tool that copies the corresponding text from the data table into the model’s response.
“This way, we know it is a verbatim copy, so we know there will not be any errors in the part of the text that corresponds to the actual data variable,” Shen adds.
Streamlining validation
The model can create symbolic responses because of how it is trained. Large language models are fed reams of data from the internet, and some data are recorded in “placeholder format” where codes replace actual values.
When SymGen prompts the model to generate a symbolic response, it uses a similar structure.
“We design the prompt in a specific way to draw on the LLM’s capabilities,” Shen adds.
During a user study, the majority of participants said SymGen made it easier to verify LLM-generated text. They could validate the model’s responses about 20 percent faster than if they used standard methods.
However, SymGen is limited by the quality of the source data. The LLM could cite an incorrect variable, and a human verifier may be none-the-wiser.
In addition, the user must have source data in a structured format, like a table, to feed into SymGen. Right now, the system only works with tabular data.
Moving forward, the researchers are enhancing SymGen so it can handle arbitrary text and other forms of data. With that capability, it could help validate portions of AI-generated legal document summaries, for instance. They also plan to test SymGen with physicians to study how it could identify errors in AI-generated clinical summaries.
This work is funded, in part, by Liberty Mutual and the MIT Quest for Intelligence Initiative.
#ai#ai model#AI models#artificial#Artificial Intelligence#attention#author#cell#computer#Computer Science#Computer Science and Artificial Intelligence Laboratory (CSAIL)#Computer science and technology#conference#data#Database#deploying#Design#Electrical engineering and computer science (EECS)#engineering#finance#financial#focus#form#Forms#game#generations#generative#generative ai#Hallucination#Health
0 notes
Text
https://digitalwinacademics.com/
#python#java#datascience#banking and finance#Digitalwin Academy provides software trainings like IT courses#sap#data science#life science#banking and finance. More Details Visit Our Website : https://digitalwinacademics.com/
0 notes
Text
How AI Identifies Emerging Market Opportunities in Financial Analysis
In the fast-paced world of finance, identifying emerging market opportunities is crucial for investors, analysts, and businesses looking to maintain a competitive edge. Traditional methods of market analysis often involve extensive manual research and interpretation of data, which can be time-consuming and prone to human error. However, the advent of Artificial Intelligence (AI) is revolutionizing financial analysis by enabling quicker, more accurate identification of market trends and opportunities. Photon Insights is at the forefront of this transformation, leveraging AI to help financial professionals uncover valuable insights. This article explores how AI is reshaping the landscape of financial analysis and the role of Photon Insights in this evolution.
The Importance of Identifying Emerging Market Opportunities
Identifying emerging market opportunities is vital for several reasons:
1. Strategic Investment Decisions: Investors rely on accurate market analysis to make informed decisions about where to allocate resources. Recognizing trends early can lead to significant financial gains.
2. Competitive Advantage: Businesses that can spot emerging markets ahead of their competitors can position themselves effectively, capturing market share and driving growth.
3. Risk Mitigation: Understanding market dynamics allows firms to anticipate changes and potential risks, enabling proactive strategies to safeguard investments.
4. Innovation and Growth: Emerging markets often present unique opportunities for innovation. Identifying these trends can inspire new products, services, and business models.
Challenges in Traditional Financial Analysis
Traditional financial analysis methods face several challenges:
1. Data Overload: The sheer volume of data generated in financial markets can be overwhelming. Analysts often struggle to sift through this information to identify relevant trends.
2. Time Constraints: Financial professionals are under constant pressure to deliver insights quickly. Manual analysis can lead to delays in decision-making.
3. Subjectivity: Human bias can affect interpretation of data, leading to inconsistent conclusions and potentially flawed investment decisions.
4. Inability to Predict Trends: Traditional analysis often relies on historical data, which may not adequately reflect future market conditions.
How AI Transforms Financial Analysis
AI is redefining financial analysis by offering tools and techniques that address the challenges of traditional methods. Here are several key ways AI enhances the identification of emerging market opportunities:
1. Advanced Data Analytics
AI algorithms can process vast datasets from multiple sources, including financial reports, news articles, social media, and market data, in real-time. This capability allows analysts to identify patterns and trends that may not be evident through manual analysis.
Keyword Focus: Data Analytics, Market Trends
Photon Insights utilizes advanced data analytics tools to enable financial professionals to uncover insights quickly, facilitating timely investment decisions.
2. Predictive Analytics
By employing machine learning algorithms, AI can analyze historical data and identify factors that influence market movements. Predictive analytics can forecast potential future trends, helping analysts anticipate emerging opportunities.
Keyword Focus: Predictive Analytics, Forecasting
Photon Insights provides predictive analytics capabilities that allow financial analysts to simulate different market scenarios, enhancing their ability to make informed decisions.
3. Natural Language Processing (NLP)
NLP enables AI to interpret and analyze unstructured data, such as news articles and social media posts. By gauging public sentiment and market discussions, AI can help analysts understand market perceptions and identify emerging trends.
Keyword Focus: Natural Language Processing, Sentiment Analysis
Photon Insights integrates NLP features that help analysts gauge market sentiment, allowing them to make more nuanced assessments of market conditions.
4. Real-Time Monitoring
AI tools can continuously monitor market data, news, and social media in real-time, providing analysts with instant alerts about significant market changes. This immediacy enables prompt action in response to emerging opportunities.
With Photon Insights, financial professionals can set up customized alerts to stay informed about critical developments that may signal new market opportunities.
5. Enhanced Visualization
AI-driven data visualization tools can present complex datasets in an easily digestible format. Visual representations of data help analysts quickly identify patterns, correlations, and outliers, facilitating faster decision-making.
Photon Insights offers advanced visualization capabilities, enabling analysts to create interactive dashboards that highlight emerging trends and opportunities.
The Photon Insights Advantage
Photon Insights stands out in the field of financial analysis by providing a comprehensive suite of AI-driven tools designed to identify emerging market opportunities. Here are several key features of the platform:
1. Comprehensive Data Integration
Photon Insights aggregates data from various sources, including market data, news, and social media, providing a holistic view of market conditions. This integration enables analysts to identify emerging opportunities based on a wide array of information.
2. User-Friendly Interface
The platform features an intuitive user interface that simplifies the process of data analysis. Financial professionals can easily navigate the tools, making it accessible even for those with limited technical expertise.
3. Customizable Dashboards
Users can create customized dashboards tailored to their specific research needs. This flexibility allows analysts to focus on the most relevant data and visualizations for their market analysis.
4. Collaboration Tools
Photon Insights promotes collaboration among team members by allowing them to share insights and findings within the platform. This collaborative environment fosters a more comprehensive understanding of market dynamics.
5. Continuous Learning
The AI algorithms used in Photon Insights continually learn from new data, improving their accuracy and predictive capabilities over time. This continuous learning ensures that analysts are equipped with the latest insights and trends.
Case Study 1 — Investment Firm
One investment firm utilized Photon Insights’ NLP capabilities to assess market sentiment around a newly emerging technology sector. By quickly recognizing positive sentiment trends, timely investments were made in emerging tech startups with positive sentiment scores; as a result, this firm achieved exceptional returns from its investments.
Case Study 2 — Retail Business
One retail business used Photon Insights to keep up-to-date on consumer trends and preferences in real-time. By analyzing social media discussions and market data, they identified an increasing demand for sustainable products that allowed them to switch up their offerings and capture an attractive market niche.
Case 3 — Financial Services Company
One financial services firm utilized Photon Insights’ predictive analytics capabilities to anticipate any market disruptions caused by regulatory changes and develop proactive strategies for mitigating risks and seizing emerging opportunities in compliance-related services.
Conclusion
AI is revolutionizing financial analysis, helping professionals to recognize market opportunities faster and with greater accuracy than ever before. Photon Insights is leading this charge with its suite of AI-powered tools designed to enhance data analysis, predictive modeling, sentiment analysis, real-time monitoring and real-time alerts.
0 notes
Text
Gethsemane Enertech ERP Solution: Revolutionizing Energy Efficiency through AI, IoT & Data Science
Overview of Energy Efficiency in Ghana and Africa Energy efficiency is increasingly becoming a focal point in the energy sector, particularly in developing regions like Ghana and Africa, where energy demand is growing rapidly due to economic expansion and population growth. In these regions, energy efficiency is not just about reducing costs but also about enhancing energy security, reducing…
View On WordPress
#AI#ESG#IoT#ACEP#Africa#Climate Change#Coronavirus#Data Science#Energy Efficiency#Future of energy Conference 2024#Gethsemane Enertech ERP Solution#Ghana#GodRules#Green finance#Impact Investing#ImpactofCovid19#LoveofGod#Oil and gas#Teamwork
0 notes
Text
What is Data Science? A Comprehensive Guide for Beginners

In today’s data-driven world, the term “Data Science” has become a buzzword across industries. Whether it’s in technology, healthcare, finance, or retail, data science is transforming how businesses operate, make decisions, and understand their customers. But what exactly is data science? And why is it so crucial in the modern world? This comprehensive guide is designed to help beginners understand the fundamentals of data science, its processes, tools, and its significance in various fields.
#Data Science#Data Collection#Data Cleaning#Data Exploration#Data Visualization#Data Modeling#Model Evaluation#Deployment#Monitoring#Data Science Tools#Data Science Technologies#Python#R#SQL#PyTorch#TensorFlow#Tableau#Power BI#Hadoop#Spark#Business#Healthcare#Finance#Marketing
0 notes
Text
What is Roll Rate Analysis?
Summary: Roll rate analysis tracks account movement through delinquency stages, helping institutions forecast defaults, manage risk, and refine credit policies. It provides essential insights for effective financial management.

Introduction
Roll rate analysis, a key metric in financial risk management, tracks the movement of accounts or loans through various stages of delinquency. By answering the question, "What is roll rate analysis?" this blog aims to explore its definition, significance, and practical applications across industries.
Roll rate analysis helps businesses forecast potential risks and manage portfolios more effectively. Understanding its role is crucial for enhancing credit risk management, improving financial predictions, and making informed decisions. This blog will provide a comprehensive guide to the methodology, benefits, and challenges associated with roll rate analysis.
Read: Stable Diffusion in Machine Learning: An In-depth Analysis.
What is Roll Rate Analysis?
Roll rate analysis is a critical financial tool used to assess the movement of accounts or loans through various stages of delinquency. It measures the rate at which accounts transition from one status to another, such as from current to overdue. This analysis helps financial institutions understand how quickly and frequently accounts progress through different stages of credit risk.
Measuring Movement Through Stages
To perform roll rate analysis, financial institutions track accounts or loans as they shift through predefined stages, such as current, 30 days past due, 60 days past due, and so on.
By calculating the percentage of accounts that roll from one stage to the next, institutions gain insights into the likelihood of future delinquencies or defaults. For example, if a significant number of accounts move from 30 days past due to 60 days past due, it indicates worsening credit conditions.
Roll rate analysis thus provides valuable information for managing credit risk and making informed decisions about credit policies and reserves. It serves as an early warning system, allowing institutions to act proactively in mitigating potential losses.
Importance of Roll Rate Analysis

Roll rate analysis plays a critical role in the financial industry, serving as a key tool for managing credit risk, forecasting financial trends, and optimizing portfolio performance. By tracking how accounts or loans progress through various delinquency stages, roll rate analysis provides valuable insights that help organizations make informed decisions.
Role in Credit Risk Management: Roll rate analysis helps identify early signs of credit risk by monitoring the movement of accounts from current to delinquent status. This proactive approach enables financial institutions to adjust credit policies, set appropriate reserves, and mitigate potential losses before they escalate.
Application in Financial Forecasting: Accurate roll rate data enhances financial forecasting by predicting future delinquency rates and defaults. This predictive capability allows organizations to estimate cash flows, plan for potential credit losses, and develop strategies for managing financial stability in varying economic conditions.
Impact on Portfolio Management: In portfolio management, roll rate analysis aids in assessing the health of a credit portfolio. By understanding the rate at which accounts transition between different stages, portfolio managers can make strategic adjustments, such as reallocating resources or adjusting investment strategies, to optimize returns and minimize risk.
Overall, roll rate analysis is essential for maintaining a robust financial strategy, ensuring that organizations are well-prepared to handle credit challenges and make informed decisions that drive long-term success.
Explore: Strategies for Transitioning Your Career from Data Analyst to Data Scientist–2024.
Key Components of Roll Rate Analysis
Roll rate analysis relies on several critical components to assess the movement of accounts or loans through different stages. Understanding these elements is crucial for accurate analysis and effective risk management.
Stages or Buckets: Roll rate analysis typically categorizes accounts into distinct stages, also known as buckets. Common stages include:
Current: Accounts that are up to date with payments.
30 Days Past Due: Accounts that have missed one payment cycle.
60 Days Past Due: Accounts that are two payment cycles behind.
90 Days Past Due: Accounts that are significantly overdue, often considered high risk.
Roll Rate Formula and Calculation: To calculate roll rates, use the following formula: This formula helps determine the percentage of accounts transitioning from one stage to the next, indicating the likelihood of default or payment issues.
Data Requirements for Accurate Analysis: Effective roll rate analysis depends on high-quality, comprehensive data, including:
Account Information: Detailed records of payment history and current status.
Stage Transitions: Accurate tracking of movement between different stages.
Historical Data: Historical data for trend analysis and comparison.
Timeliness: Up-to-date data to reflect current conditions and ensure reliability.
By focusing on these key components, organizations can gain valuable insights into their portfolio’s health and proactively manage potential risks.
Discover: The Transformative Role of Data Science in Stock Market Analysis.
Benefits of Roll Rate Analysis

Roll rate analysis offers valuable insights that can significantly enhance financial management and risk mitigation strategies. By tracking the movement of accounts through different delinquency stages, roll rate analysis provides crucial information that helps organizations make informed decisions and manage their credit portfolios more effectively.
Early Warning of Potential Defaults: Roll rate analysis identifies accounts that are progressing through stages of delinquency, allowing organizations to anticipate potential defaults before they occur. This early warning system helps in taking proactive measures to mitigate risks, such as adjusting credit limits or initiating collection processes earlier.
Improved Risk Management: By monitoring the roll rates, companies gain a better understanding of their portfolio's risk profile. This enables them to identify trends and patterns in delinquency, allowing for more accurate risk assessments and adjustments in credit policies. Enhanced risk management ultimately reduces the likelihood of significant financial losses.
Enhanced Decision-Making for Credit Policies: Roll rate analysis provides actionable data that supports more informed decision-making regarding credit policies. Organizations can refine their credit criteria, adjust interest rates, or develop targeted interventions based on the observed roll rates. This leads to more effective credit management and improved overall financial stability.
Challenges in Roll Rate Analysis
Roll rate analysis offers valuable insights into account movement and credit risk, but it comes with several challenges that can impact its effectiveness. Understanding these challenges is crucial for accurate and actionable analysis. Here’s a closer look at the main obstacles:
Data Quality Issues: Accurate roll rate analysis heavily relies on high-quality data. Incomplete, outdated, or erroneous data can lead to misleading results. Ensuring data integrity involves regular validation and cleaning processes. Inaccurate data can distort roll rate calculations, affecting risk assessments and decision-making.
Model Limitations: Roll rate models often depend on historical data to predict future performance. However, these models might not account for all variables or changes in the economic environment. Over-reliance on historical trends can limit the model’s ability to adapt to new patterns or unforeseen events, leading to less reliable forecasts.
Adapting to Changing Economic Conditions: Economic conditions can significantly impact roll rates. Changes in the economy, such as recessions or shifts in interest rates, can alter the behavior of account holders. Roll rate analysis models must be flexible and regularly updated to reflect these changes. Adapting to economic fluctuations requires continuous monitoring and adjustment of models to maintain accuracy.
Addressing these challenges involves improving data management practices, refining analytical models, and staying responsive to economic changes. By tackling these issues, organizations can enhance the reliability and usefulness of their roll rate analysis.
Check: Is Python a Scripting Language? A Technical Analysis.
Frequently Asked Questions
What is roll rate analysis used for?
Roll rate analysis is used to track the movement of accounts through different stages of delinquency. It helps financial institutions assess credit risk, forecast potential defaults, and manage credit portfolios effectively.
How is roll rate analysis calculated?
Roll rate analysis is calculated by determining the percentage of accounts transitioning from one delinquency stage to another. For example, it measures how many accounts move from 30 days past due to 60 days past due.
What are the benefits of roll rate analysis?
Roll rate analysis provides early warnings of potential defaults, enhances risk management, and supports informed decision-making for credit policies. It helps organizations anticipate issues and optimize their credit strategies.
Conclusion
Roll rate analysis is a vital tool for managing financial risk by tracking the progression of accounts through various delinquency stages. It enables institutions to forecast potential defaults, refine credit policies, and optimize portfolio management. Despite challenges like data quality and model limitations, effective roll rate analysis provides valuable insights for proactive credit risk management.
#What is Roll Rate Analysis?#Roll Rate Analysis#data analyst#Data analysis#finance#financial analysis#data science#risk management#financial risk#financial risk management#portfolio management
0 notes
Text
Data Science in Finance: Predictive Modeling and Risk Management
Data Science in Finance: Predictive Modeling and Risk Management involves using statistical techniques and machine learning to predict financial trends and assess risk. By analyzing large datasets, financial institutions can forecast market movements, identify potential risks, and make data-driven decisions to optimize portfolios, enhance investment strategies, and ensure regulatory compliance.
Read More: https://blognow.co.in/data-science-in-finance-predictive-modeling-and-risk-management
0 notes
Text
The Impact of Big Data Analytics on Business Decisions
Introduction
Big data analytics has transformed the way of doing business, deciding, and strategizing for future actions. One can harness vast reams of data to extract insights that were otherwise unimaginable for increasing the efficiency, customer satisfaction, and overall profitability of a venture. We steer into an in-depth view of how big data analytics is equipping business decisions, its benefits, and some future trends shaping up in this dynamic field in this article. Read to continue
#Innovation Insights#TagsAI in Big Data Analytics#big data analytics#Big Data in Finance#big data in healthcare#Big Data in Retail#Big Data Integration Challenges#Big Data Technologies#Business Decision Making with Big Data#Competitive Advantage with Big Data#Customer Insights through Big Data#Data Mining for Businesses#Data Privacy Challenges#Data-Driven Business Strategies#Future of Big Data Analytics#Hadoop and Spark#Impact of Big Data on Business#Machine Learning in Business#Operational Efficiency with Big Data#Predictive Analytics in Business#Real-Time Data Analysis#trends#tech news#science updates#analysis#adobe cloud#business tech#science#technology#tech trends
0 notes
Text
0 notes